ChatGPT’s debut in November 2022 caused a near-instant sensation. It gave most users their first opportunity to try out a new type of artificial intelligence that uses existing data and published materials to create content known as generative AI.
Once the initial buzz faded, economists were eager to find out who was using the technology and how often, what they were doing with it, and whether they used it at work, at home, or both. How quickly and robustly the public adopts a technology is widely thought to predict its economic impact.
As of August, nearly 40 percent of U.S. adults aged 18-64 had used generative AI, according to new survey research. Of those employed, 28 percent used it at work, while nearly 33 percent used it away from work. That pickup rate is significantly faster than the public embrace of the internet (20 percent after two years) or the personal computer (20 percent after three years, the earliest researchers could measure).
The Gazette spoke with David J. Deming, the Isabelle and Scott Black Professor of Political Economy at Harvard Kennedy School, and professor of economics and education at Harvard Graduate of Education, about what he and co-authors Alexander Bick, an economic policy adviser at the Federal Reserve Bank of St. Louis, and Adam Blandin, assistant economic professor at Vanderbilt University, found and what it could mean for business. Interview has been edited for clarity and length.
Why is it important to measure how quickly Americans have embraced generative AI tools like ChatGPT relative to PCs and the internet?
For a new technology like this, it’s really important for us to have some baseline understanding of how much it’s used and by whom, and what are they using it for. To do that, you need a high-quality, nationally representative survey. So, we recreated all of the question wording and the structure of the Current Population Survey (CPS), which is the big survey that produces the unemployment rate every month. It’s the main source of labor market data in the U.S.
The CPS, back in 1984, started asking questions about usage of the personal computer at home. In 2001, it started asking questions about internet usage. And so, we took the same question ordering, replacing those technologies with generative AI, so we could directly compare it to the speed of adoption of other technologies, asking the same people the same questions.
That’s how we are able to show the usage rate in our data, which is 39.4 percent, is actually higher than both personal computers and the internet at the same stage in their product cycles.
In which specific tasks is AI most useful at work?
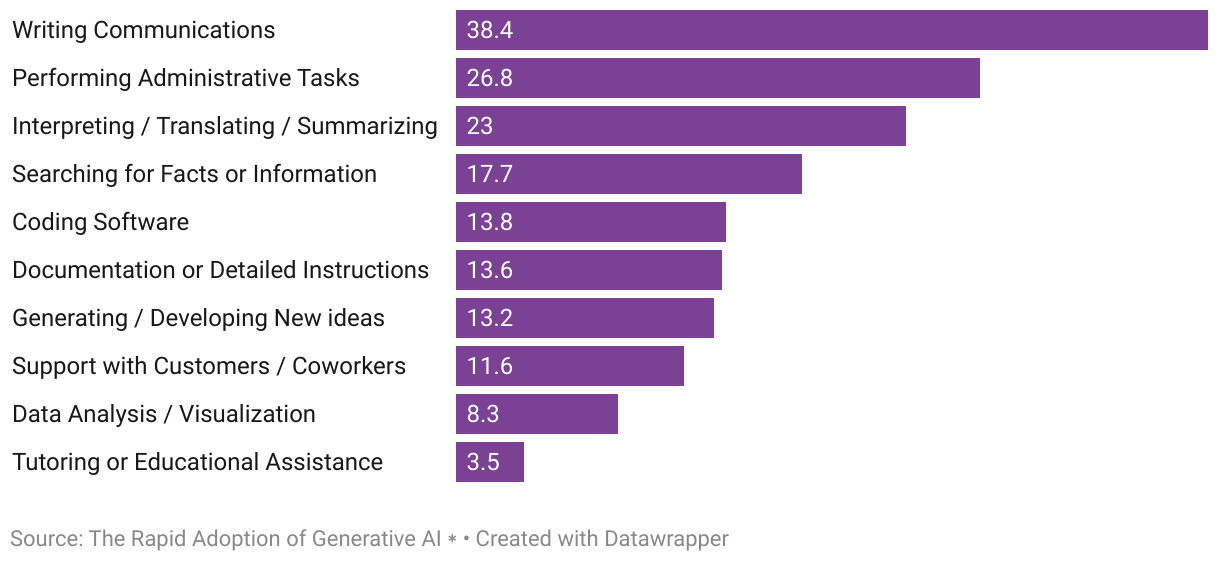
In which specific tasks is AI most useful at home?
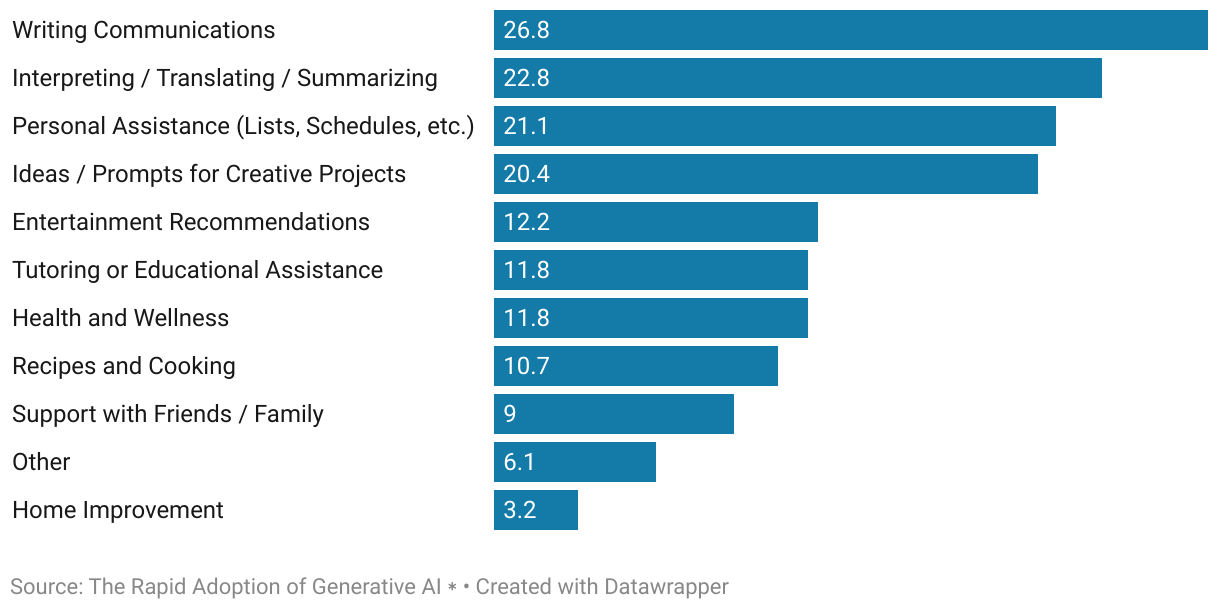
Were you and your team surprised by these findings?
I personally was surprised at the high rate of usage. Whenever I tell colleagues about this, I always ask, “What do you think we found?” before I tell them. Most of my colleagues are aging academics like me, so we tend to underestimate generative AI usage. When I ask my graduate students or undergraduates, they tend to estimate numbers that are higher than the actual number we found. And I think that really tells you something about it. We found that young people are using generative AI at much, much higher rates than older people, which is very common across other technologies.
I didn’t come into this thinking this is what we’re going to find. I just was interested in the answer because I’d read a lot of things suggesting it was mostly hype, and I read a lot of things suggesting it was the Next Big Thing. And so, we wanted to know where the truth was.
What accounts for such swift adoption?
I can give you some informed speculation. One is that generative AI is built on top of those previous two technologies. You could think about the fact that people have computers in their home, and they have access to the internet, as base layers that allow you to easily adopt some new technology like generative AI. The personal computer, when it was released, was big and expensive and not everyone had it at home. The internet was less expensive, but we built this incredible grid that allowed people to be connected. Without those two things, you wouldn’t have generative AI. I think one of the reasons why it’s been adopted quickly is because the base level technologies were already there, and you could, in some sense, think about generative AI as a complementary innovation to the internet.
Demographic differences in AI use at work
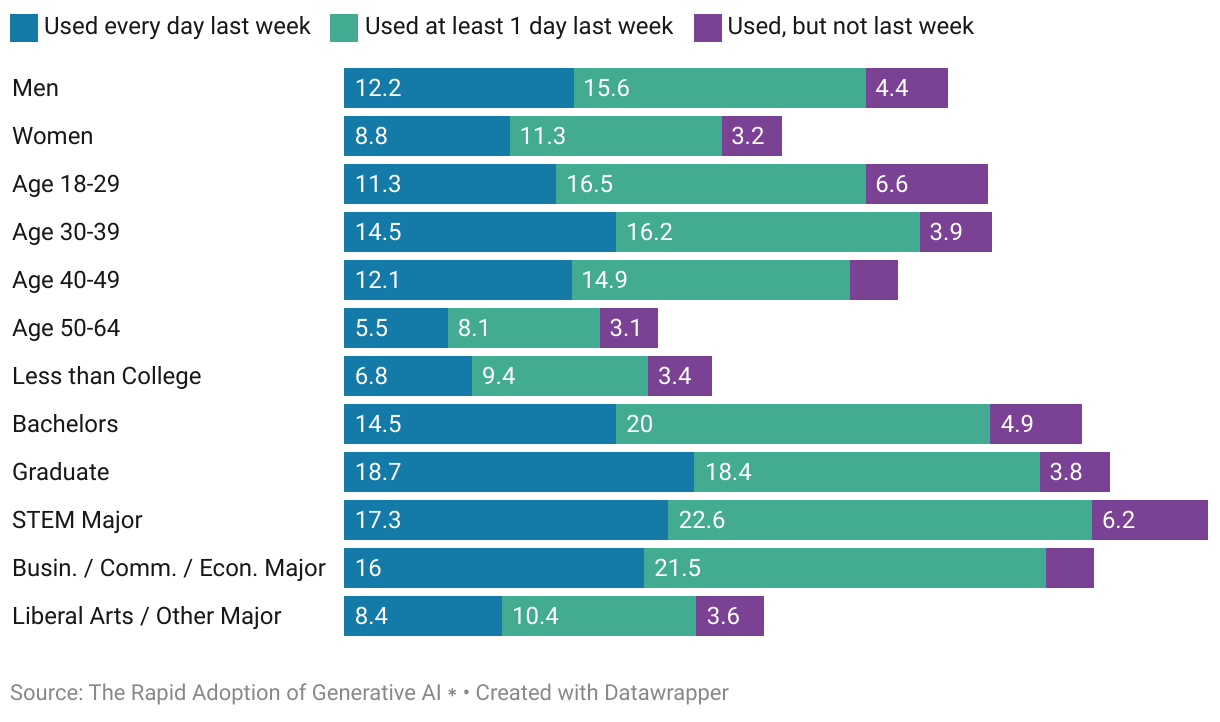
Adoption is not uniform across demographic groups. Men, younger people, those with a college or graduate school education, and people in white-collar jobs are more likely to use generative AI and more often. What accounts for the usage gaps?
The bit about younger people and more educated people adopting a technology is actually common to almost every new technology. In studies of the adoption of personal computers, people found the same thing. The one thing that was different, relative to PCs, was gender. Women used PCs at work more than men in the 1980s largely because the job of administrative assistant or secretary, and office jobs in general, skewed very female and they were using computers whereas generative AI is not as concentrated in occupations. It’s everywhere. We find higher usage in STEM and management careers and those do skew male.
I don’t think access is explaining it because a lot of people use computers on the job. We found very, very broad adoption across occupations. It’s highest in STEM jobs and management, but 22 percent of blue-collar occupations were using AI. And usage rates were above 20 percent in every category of occupations except personal services, so it’s really common across places.
If you look at how many companies say they’re using it, it’s actually a pretty low share who are formally incorporating it into their operations. People are using it informally for a lot of different purposes, to help write emails, using it to look up things, using it to obtain documentation on how to do something. I think a lot of variation reflects the fact that some people like to tinker, and companies aren’t telling you don’t use it, but they’re not necessarily formally requiring you to use it.
This is something we’re really interested in tracking. We’ve already started our discussions about the next wave, and we’ll try to update the data over time and ask more questions about usage and dig into some of the threads that were left hanging.
AI use at work by occupation
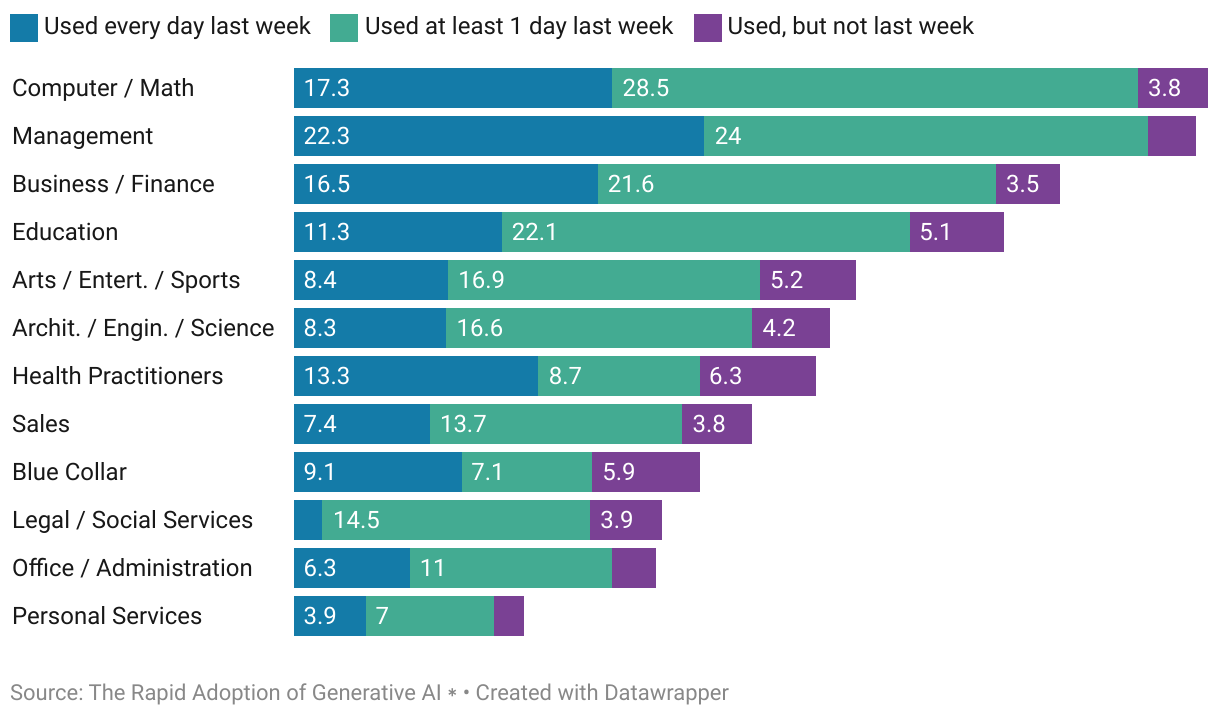
AI use at work by industry group
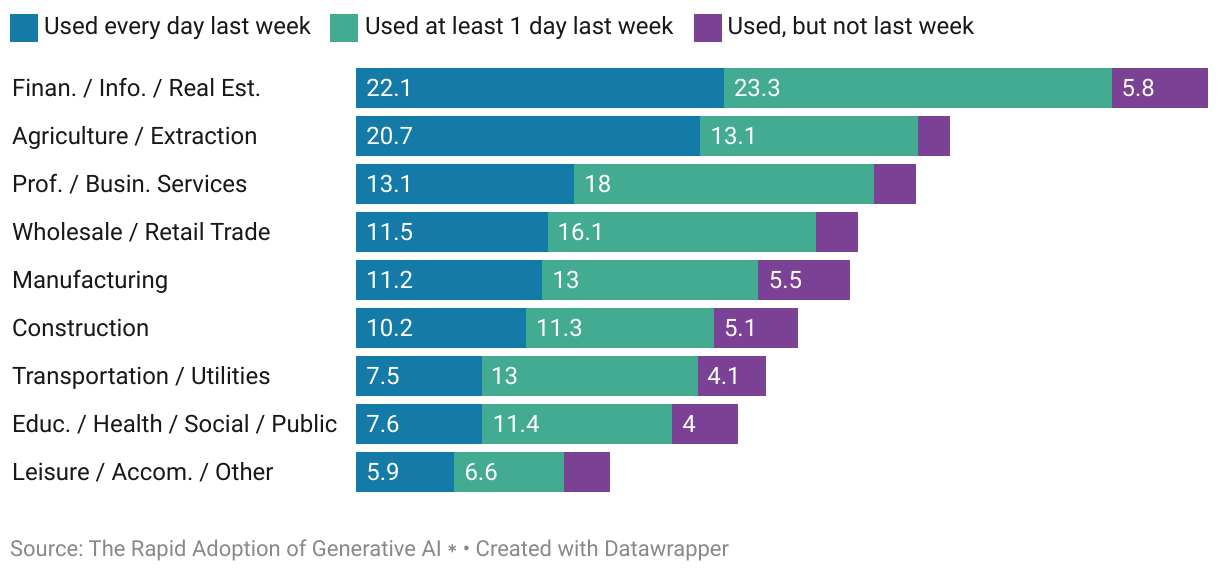
This is the first-ever national survey on this subject. Should business and tech executives consider acting on any of these findings?
I would say most definitely yes. Another way to think about this is if you were to go back to 1984 and tell people, “Hey, there’s this new thing called the personal computer. I have a crystal ball. Twenty years from now, everybody’s going to have one of these and every single new technological development and every single new product is going to be using it as the base.” Knowing that now, what would you do differently? You would change a lot. You could make billions and billions of dollars.
I think this survey is saying, “We don’t have a crystal ball, but it sure looks like generative AI is going to be on that scale.” And so, the spoils will go to people who can figure out how to harness it first and best. So yes, I think they should be paying attention. I think a lot of them are already.
Much like the internet was a base layer for a lot of other technologies, you’ll see that the people who figure out how to use this technology that’s so versatile, that can do so many things well, but doesn’t yet have a killer app — the people who find that killer app, who build something on top of it, are going to really, really profit and benefit. I think that’s what the next five or 10 years will be about.
These companies are about trying to build human-level intelligence. That’s fine, but there are a lot of commercial applications that don’t require that. They need to be built with this thing as an input into it, rather than this being the product itself. So, I think you’ll see a lot of that in the next few years, and it’s going to be really exciting.
Source link